Environmental Remote Sensing and Data Analytics (ERSA) Lab is designed as the central research theme to understand how the world is changing based on quantitative remote sensing. The research uses a variety of remote sensing sensors such as infrared, thermal infrared and microwave radiometers, gamma sensors, drones, to estimate hydro-meteorological parameters, monitor environmental change at local level, and develop algorithms at regional to global scales. The lab is committed to providing research and growth opportunities in STEM.
Data Science and Artificial Intelligence/Machine Learning
Scientific methods using complex machine learning and data modeling in Remote Sensing (RS) are increasing and evolving owing to the growing impact of information technology and data science advancements in extracting insights from data. This data deluge is available through open data/clouds. Open Science is pioneered by the provision and implementation of open data, data policy of RS data, and data products. Data science technology links complex spatial data taken from local in-situ measurements to the regionally and globally-linked data. The reasoning behind this is that free access to data (Open Data) leads to extensive gaining of information that further helps understand complex patterns and multidimensional processes in ecosystems, the complexity of our landscape and the modeling the landscape consists of. This lab uses the observed data sets derived from various research facilities that are operated by CUNY CREST, data from NOAA, and the NASA Satellite data. Further, data science tools that help us extract important information through data modeling help the decision makers.
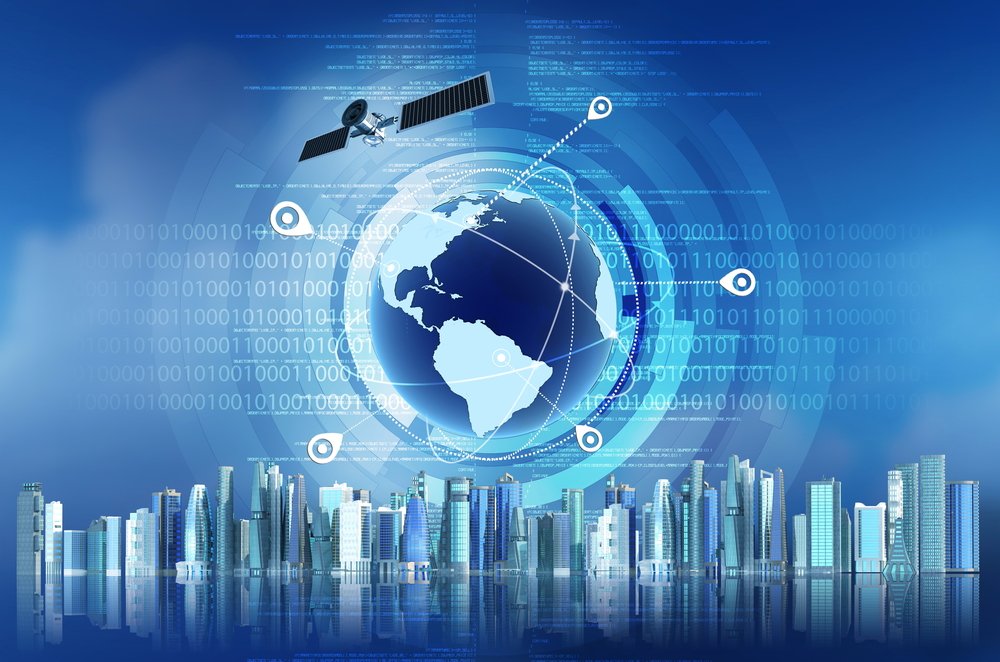
Snow Remote Sensing
Since 2010, the CUNY-Snow Analysis and Field Experiment (CUNY-SAFE) is conducted each winter. This experiment builds on earlier studies by extending over the full winter season instead of only one or a few days, thus allowing processes of snowpack accumulation, metamorphism, and melting – and their impacts on microwave emissivity – to be observed sequentially at the same site. The objective of this long term field experiment is to characterize the behavior of snow-emitted microwave radiation throughout the winter season. The experiment examines and interprets the effect of snowpack temperature (related to dry and wet snow conditions) on the microwave brightness temperature. The continuation of long-term observations of in situ snow properties combined with microwave radiances will facilitate the assessment of current snow retrieval algorithms and their capability to infer snow properties under different meteorological conditions. https://www.cessrst.org/snow/
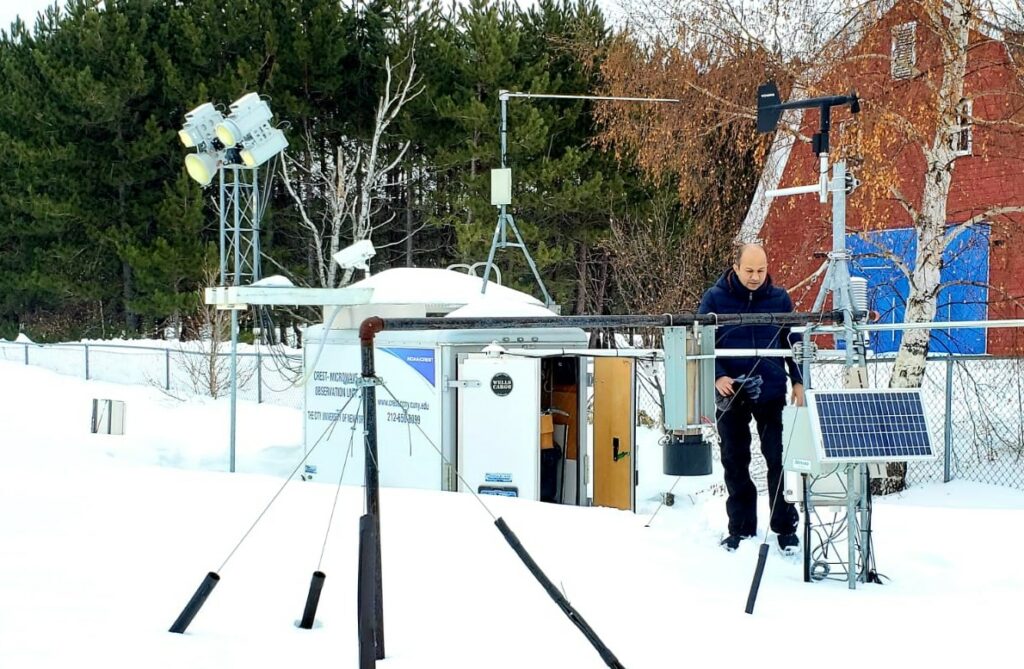
Urban Climate and Social Vulnerability
Monitoring micro-climate variables within cities that have a high accuracy for a better urban resilience to climate change is an ongoing challenge. Assessing the intra-urban characteristics of a city is vital to ensuring better living standards. Urban meteorological networks and automated data acquisition is highly needed for modern urban climate monitoring, evaluation, and analysis. Following research questions are being investigated using NY-uHMT datasets: How does incorporation of urbanization data into the flash flood guidance system by creating the static grid/pixels help identify and isolate areas where land development has altered the runoff characteristics in NYC?; What role does soil moisture deficit between the urban and surrounding rural areas play in exacerbating urban air temperature? And how does it impact the micro-climate of the cities: local and regional?; Can integration of ground based in-situ observation with radar and urbanized Weather Research and Forecasting (uWRF) model (used to understand the spatial and temporal distribution of heavy rainfall that are influenced by urban heat island, urban canopy and urban aerosols) be updated by higher resolution soil moisture records? https://www.cessrst.org/uHMT/
Remote Sensing of Soil Moisture
CREST-SMART (Soil Moisture Advanced Radiometric Testbed) field campaign aims to improve microwave radiative modeling of soil–vegetation systems and improve the understanding of soil–plant–atmosphere interactions. It consists of two components: (i) microwave remote sensing using L-band radiometry, (ii) a comprehensive network of ground measurements of soil moisture. The objectives of campaign are: (i) to contribute towards a better understanding of the different processes affecting the microwave signal, (ii) to test as well as improve the soil moisture retrieval algorithms from dual polarized and multi-angular measurements, and (iii) to validate satellite based soil moisture products against in situ observations. https://www.cessrst.org/smart/
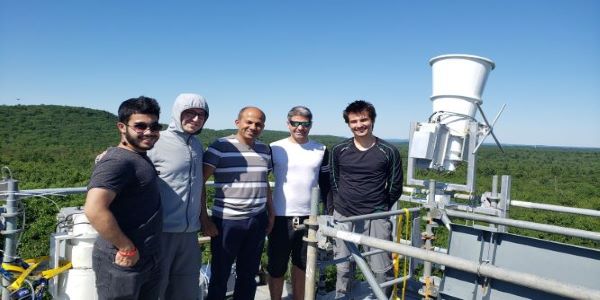
Remote Sensing of Solar Energy
Information about solar radiation (affected by clouds and haze) is important for managing solar power systems, among other applications. This facility will compare re-analysis and satellite based surface solar radiation products with station measurements to better understand their relative accuracy. It may also experiment with different approaches for short-term forecasting of solar radiation and work towards setting up ground network sites to validate solar radiations in Puerto Rico. Presently, CUNY CREST is setting up facilities to conduct solar radiation based experiments in Puerto Rico.